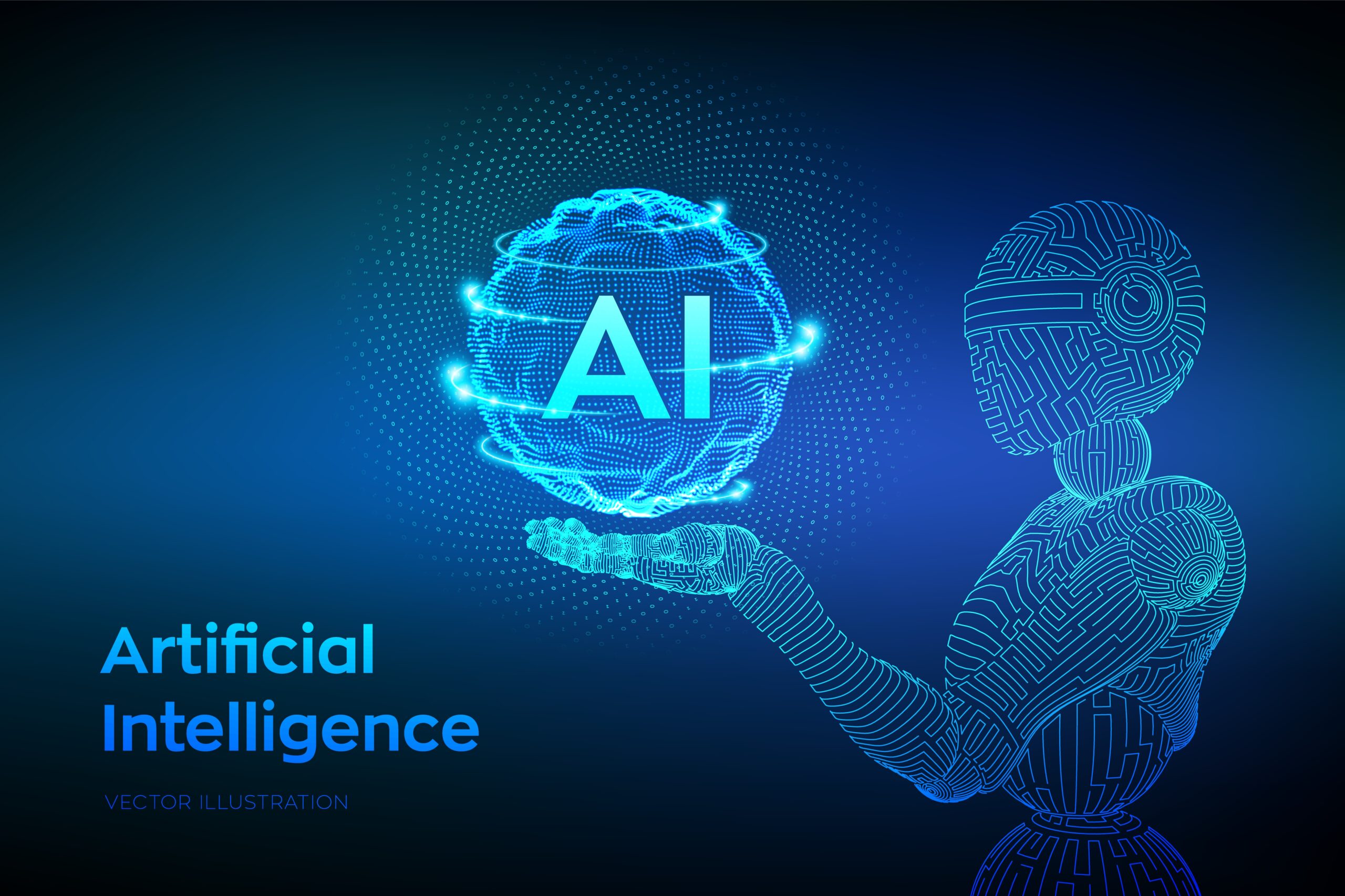
"Technical and ethical challenges of implementing AI based solutions in real environments"
Challenges and New Developments Towards Smarter, Safer, More Reliable, and More Resilient Autonomous Transportation and Renewable Energy Systems
Research on Fault Detection and Diagnosis (FDD) and Fault-Tolerant Control (FTC) has been ongoing since the 1970s, but recent accidents highlight their renewed importance. These techniques ensure the safety and security of complex systems like Smart Grids (integrating renewable energy) and Unmanned Systems (drones, self-driving cars).
Advances in materials, communication, and AI benefit these autonomous systems (AS). However, their complexity and reliance on electronics make them susceptible to physical faults and cyberattacks, which can compromise stability, performance, safety, and security.
This talk will discuss challenges and advancements in FDD, FTC, and Fault/Attack-Tolerant Cooperative Control (FTCC) for safe and secure operation of Smart Grids and Unmanned Systems in tasks like forest fire monitoring.
About the speaker:
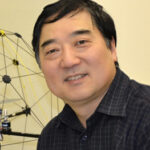
Youmin Zhang
Department of Mechanical, Industrial & Aerospace Engineering & Concordia Institute of Aerospace Design and Innovation (CIADI), Concordia University
Dr. Youmin Zhang (IEEE Fellow) is currently a Professor at the Department of Mechanical, Industrial and Aerospace Engineering at Concordia University, Canada. His research interests include condition monitoring, health management, physical-fault and cyber-attack detection, diagnosis and tolerant/resilient control, cooperative guidance, navigation and control of unmanned aerial/space/ground/marine vehicles, smart grids, and smart cities under the framework of Cyber-Physical Systems (CPSs). He has published 10 books, over 600 journal and conference papers, and book chapters. He is a Fellow of IEEE and Canadian Society of Mechanical Engineering (CSME), President of International Society of Intelligent Unmanned Systems (ISIUS, 2019-2022). He has been an (Deputy) Editor-in-Chief, Associate Editor, (Advisory) Editorial Board Member of more than 20 journals. He has served as (Honorary) General Chair, Program Chair of several autonomous/unmanned systems, renewable energies, and smart cities relevant international conferences. He has been ranked #1 worldwide on “Aircraft systems” and “Fault tolerance” (Lifetime); #1 in Canada and #6 worldwide on “Unmanned aerial vehicle” (Lifetime) in the recent ranking at ScholarGPS™ by 2024 Meta Analytics LLC. More detailed information can be found at http://users.encs.concordia.ca/~ymzhang/.
Predictive control: basic concepts, applications, advances and challenges
Model Predictive Control (MPC) emerged years ago as an attractive control strategy for power electronics systems. The main advantages of MPC are its conceptual simplicity, the ability to include various control objectives, and high dynamic performance. On the contrary, like any new strategy, it has disadvantages such as mathematical model dependence, parameter dependence, and a variable frequency spectrum. However, thanks to the work carried out by the scientific community, most of these problems have been solved. This talk introduces the audience to the MPC and its evolution and applications. Special attention will be paid to using MPC in multilevel inverters using a few calculations and avoiding the use of weight factors. The presentation will also present the evaluation used in high-power inverters and electric vehicles. Finally, this talk will discuss the challenges that MPC must overcome to be adopted by the industry.
About the speakers:
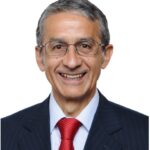
José Rodriguez Pérez
Universidad San Sebastián de Santiago de Chile
José Rodríguez is a Civil Electrical Engineer from the Federico Santa María Technical University, Chile, and a Doctor Ingenieur from the University of Erlangen, Federal Germany. His research has been dedicated to the control and transformation of electrical energy. He is the author of +900 publications, +400 in mainstream journals, +80,000 citations, and a Hirsch factor of H = 113. He is the only researcher in South America who has been included uninterruptedly between the years 2014 and 2023 on the list of “Highly Cited Researchers” that recognizes the most influential authors in the world. In 2014 he obtained the National Prize for Applied and Technological Sciences from the Government of Chile. He is a Life Fellow of the IEEE and a Chilean Academy of Engineering member. He has been Rector of the Federico Santa María, Andrés Bello and San Sebastián Technical Universities in Chile.
Artificial Intelligence Techniques for Solving Problems in the Electrical Sector
This conference titled delves into the transformative role AI plays in modernizing and optimizing the electrical industry. Participants will explore advanced AI methodologies, including machine learning, predictive analytics, and optimization algorithms, tailored specifically to address challenges within the electrical sector. Key topics will include the integration of AI for predictive maintenance, fault detection, energy efficiency, and demand forecasting. The conference will also showcase case studies demonstrating successful AI implementations in real-world electrical systems, emphasizing the potential for increased reliability, cost savings, and sustainability. Attendees will gain insights into the latest AI technologies and their practical applications, learning how to leverage these tools to enhance decision-making processes and improve operational efficiency. This event is ideal for engineers, data scientists, and industry professionals seeking to stay at the forefront of technological innovation in the rapidly evolving electrical sector.
About the speaker:
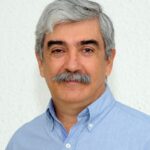
Pablo H. Ibargüengoytia
Distinguished Visitor of the IEEE CS Latin America
Pablo Ibargüengoytia holds a degree in Electronic Engineering from the Universidad Autónoma Metropolitana, a Master of Science from the University of Minnesota, USA, and a PhD in Computer Science from the University of Salford, Great Britain, specializing in Artificial Intelligence. He joined the Instituto de Investigaciones Eléctricas (IIE) in 1983, now the Instituto Nacional de Electricidad y Energías Limpias (National Institute of Electricity and Clean Energies). In 1994, he began his doctoral studies at the University of Salford in England. Since then, he has been dedicated to developing artificial intelligence applications in energy. From 1998 to 2013, he was a Professor at the Tecnológico de Monterrey, Cuernavaca campus, with the Intelligent Systems course. In 2015 and 2016, he taught a class of artificial intelligence applications applied to renewable energies at the Institute of Renewable Energies (IER) of UNAM. He is the author of national and international articles. He is a member of the Mexican Society of Artificial Intelligence (SMIA), Senior Member of IEEE, the Mexican Academy of Computing (AMEXCOMP), and the Academy of Sciences of Morelos (ACMor). He retired from the institute in August 2019 and since then founded the technology-based company Naatik A.I. Solutions to develop artificial intelligence applications in companies and industries in the country.
System Identification: An overview of recent directions with examples in discrete-state system identification
Classic system identification methods focus on identifying continuous-valued dynamical systems from input-output data, where the main analysis of such approaches largely focuses on asymptotic convergence of the estimated models to the true models, i.e., consistency properties. More recent identification approaches have focused on sample complexity properties, i.e., how much data is needed to achieve an acceptable model approximation. In this talk we will give a brief overview of classical methods and then discuss more recent data-driven methods for modeling continuous-valued linear systems and discrete-valued dynamical systems evolving over networks. Examples of the latter systems include the spread of viruses and diseases over human contact networks, the propagation of ideas and misinformation over social networks, and the spread of financial default risk between banking and economic institutions. In many of these systems, data may be widely available, but approaches to identify relevant mathematical models, including underlying network topologies, are not widely established or agreed upon. We will discuss the problem of modeling discrete-valued, discrete-time dynamical systems evolving over networks, and outline analysis results under maximum likelihood identification approaches that guarantee consistency conditions and sample complexity bounds. Applications to the aforementioned examples will be further discussed as time allows.
About the speaker:
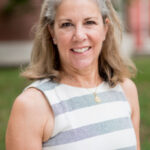
Carolyn Beck
University of Illinois at Urbana-Champaign in the Industrial and Systems Engineering department
Carolyn Beck is currently a Professor and Associate Head at the University of Illinois at Urbana-Champaign in the Industrial and Systems Engineering department. Carolyn completed her PhD at Caltech (1996), her MS at Carnegie Mellon (1985), and her BS at California State Polytechnic University (1984), all in Electrical Engineering. Prior to her PhD studies, she worked as a Research and Development Engineer for Hewlett-Packard in Silicon Valley (1985-1989). Carolyn has held visiting academic positions at KTH (Stockholm, Sweden, 2013), Stanford University (2006), and Lund University (Sweden 1996). She is currently the President-Elect for the IEEE Control Systems Society (CSS) and serves on the IEEE CSS Board of Governors. Carolyn has been elected an IEEE Fellow and is currently an IEEE CSS Distinguished Lecturer. She was the past recipient of a NSF CAREER Award, an ONR Young Investigator Award, and local teaching and advising awards. Her main research interests lie in the general area of control theory, with focused interests in the development of model identification and approximation methods, network inference, and distributed optimization and control, with applications to epidemic processes, and energy networks.
Technology-Enhanced Assessment Systems: Opportunities and Challenges
Advances in educational assessment, cognitive science, artificial intelligence, and educational technology have made it possible to create technology-enhanced learning and assessment environments that provide teachers with relevant information to support instruction and students with opportunities to learn and develop skills in a variety of areas including science inquiry, critical thinking, argumentation, and collaborative problem solving. It is expected that future learning and assessment systems will pay more attention to the context in which learning takes place. In this talk, I will discuss some of the affordances and challenges associated with the adoption of various types of educational innovations including personalized learning and assessment systems, conversation-based assessment, caring assessments, game-based assessment, and interactive dashboards. The importance of applying a principled design framework will be highlighted. This framework can help with processes such as evidence identification, evidence aggregation, and interpretability of assessment claims. The implementation of an open evidence layer in modern learning and assessment systems will be suggested as a mechanism for supporting explainability and adoption of these AI-based systems.
About the speaker:
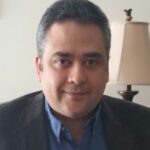
Diego Zapata-Rivera
Diego Zapata-Rivera is a Distinguished Presidential Appointee at the ETS Research Institute, specializing in technology-enhanced assessments and personalized learning environments
Diego Zapata-Rivera is Distinguished Presidential Appointee at Educational Testing Service in Princeton, NJ. He earned a Ph.D. in computer science (with a focus on artificial intelligence in education) from the University of Saskatchewan in 2003. His research at ETS has focused on the areas of innovations in communicating assessment results to various audiences and technology-enhanced assessment including work on personalized learning and assessment environments, conversation-based assessment, caring assessment, and game-based assessment. His research interests also include Bayesian student modeling, open student models, virtual environments, authoring tools, and program evaluation. Dr. Zapata-Rivera has produced over 140 publications including edited volumes, journal articles, book chapters, and technical papers. He has served as a reviewer for several international conferences and journals. He has been a committee member and organizer of international conferences and workshops in his research areas. Dr. Zapata-Rivera was elected as a member of the International AI in Education Society Executive Committee (2022-2027). He is a Co-PI and research co-director of a new NSF AI Institute – the INVITE institute (invite.illinois.edu) and was the PI of the methods and measurement project funded by EF+Math/AERDF (2019-2023). He is a member of the Editorial Board of User Modeling and User-Adapted Interaction, Associate Editor for AI for Human Learning and Behavior Change, and former Associate Editor of the IEEE Transactions on Learning Technologies Journal. Dr. Zapata-Rivera has been invited to contribute his expertise to projects sponsored by the National Research Council, the National Science Foundation, NASA
Efficient deep learning computations and its applications
Deep learning can provide superior performance in many fields of applications. However, the cost of implementing deep learning models in practical applications is expensive. Deep learning models are both computation intensive and memory intensive. Computation is an important aspect for deep learning. It can determine the latency that is how fast the results can be obtained. In this seminar, computer arithmetic for deep learning will be discussed. This lecture will start with discussing the computation requirements of deep learning models and layers. Then, several computer arithmetic designs for deep learning in the literature will be used as examples. Finally, future trends of computer arithmetic for deep learning computation will be discussed. Posit is designed as an alternative to IEEE 754 floating-point format for many applications. It has non-uniformed number distribution, and it can provide a much larger dynamic range than IEEE floating-point format. These make posit especially suitable for deep learning applications. In recent years, more and more posit based deep learning hardware accelerators appear in the literature. In this lecture, the basics of posit format and the corresponding posit-based arithmetic units available in the literature, including adder, multiplier, multiply-accumulate unit, and quire operator, will be discussed. Then, several posit-based deep learning processors for deep learning inference and training will be discussed. Finally, the trends and challenges of posit arithmetic units and posit based deep learning processors will be discussed to motivate more related research works.
About the speaker:
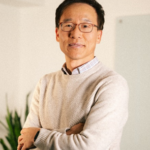
Seokbum Ko
Distinguished Lecturer of the IEEE Circuits & Systems Society, Professor at the Department of Electrical and Computer Engineering and the Division of Biomedical Engineering, University of Saskatchewan, Canada
Seokbum Ko is currently a Professor at the Department of Electrical and Computer Engineering and the Division of Biomedical Engineering, University of Saskatchewan, Canada. He received his PhD from the University of Rhode Island, USA in 2002. His areas of research interest include computer architecture/arithmetic, efficient hardware implementation of compute-intensive applications, deep learning processor architecture and biomedical engineering. He is an IEEE Cicuits and Systems Society Distinguished Lecturer (2024-2025), a senior member of IEEE circuits and systems society and an associate editor for IEEE TVLSI, IEEE TCAS-II, IEEE Access and IET Computers & Digital Techniques. He is an active member of IEEE CAS Technical Committee, IEEE P3109, IEEE754-2029, IEEE Domain-Specific Accelerators Standarads Committee and IEEE Emerging Processor Systems Standards Committee. He was an associate editor for IEEE TCASI (2019-2021).
Light works with robots
This presentation will cover how robotics is used to automate repetitive tasks in industrial production, enhancing efficiency and safety. We will focus on collaborative robots (Cobots), which work alongside humans to take over repetitive or hazardous tasks. These solutions are increasingly being adopted in industries to improve productivity and reduce costs.
About the speaker:
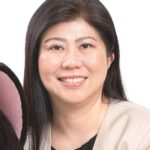
Yukiko Nakagawa
RT Corp
In 2005, she started RT Corporation, a company that develops, sells, and trains university students and professional engineers about service robots, and became its representative director. RT is the official ROS supporter of Denso Wave COBOTTA. RT is also a Jetson Education Partner. Concerning Google ADK production and sales, RT became the first Japanese company to be introduced at the Google I/O in 2011. In 2015, she was selected as one of the “25 Women in the World You Should Know in the Robotics Industry” by ROBOHUB in Silicon Valley, USA. In 2017, the company received investment from the Mirai Sousei Fund as a lead investor to develop Foodly, a humanoid collaborative robot for food factories. It is also attracting attention as a rapidly growing venture. She has published many papers and books, and writes a column for robotics or food magazines. She is also the executive director of the New Technology Foundation, which organizes Micromouse, a maze analysis robot competition, and a board member of ROSConJP, a general incorporated association. In 2020, she received the Strait of Magellan Award. In 2022, she was certified as a fellow of robot education and food robotics from the Robotics Society of Japan.
About the speaker:
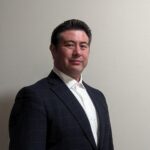
Daniel Hiroshi Neagari
RT Corp
Daniel Hiroshi Neagari is a seasoned professional with extensive international experience in business development, sales, and trade relations. Fluent in Japanese, Spanish, and English, he has worked in key roles within Japan, including his current position in international sales at RT Corporation, where he establishes new client relationships in both academic and industrial sectors. Previously, Daniel served as a commercial advisor for the Chilean and Colombian trade commissions, where he produced reports on bilateral commerce, agricultural affairs, and food safety. His expertise includes managing relationships with ministries, public organizations, and industries, facilitating international collaborations, and supporting the promotion of products and services. With a background in marketing, project coordination, and strategic planning, Daniel has also worked on projects across various countries, including Chile, Panama, Pakistan, Kenya, and Malawi. His multicultural skills and ability to navigate complex trade environments make him a valuable asset in international business and commerce.